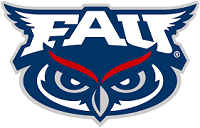
New AI technique significantly increases Medicare fraud detection
FAU’s engineering study explores the “vast sea” of Big Medicare Data, Parts B and D
Medicare is sporadically compromised by fraudulent insurance claims. These illicit activities often go undetected, allowing full-time criminals and unscrupulous healthcare providers to exploit weaknesses in the system. Last year, estimated annual fraud topped $100 billion according to the National Health Care Anti-Fraud Association, but it’s likely much higher.
Traditionally, to detect Medicare fraud, a limited number of auditors or investigators are tasked with manually inspecting thousands of claims, but they only have enough time to look for very specific patterns that indicate suspicious behavior. Also, there aren’t enough investigators to keep up with the various Medicare fraud schemes.
Using big data, such as patient records and provider payments, is often considered the best way to produce effective machine learning models to detect fraud. However, in the field of Medicare insurance fraud detection, handling large, unbalanced and high-dimensional data, data in which the number of features is surprisingly high, so that the calculations become extremely difficult, remains a major challenge.
New research from Florida Atlantic University’s College of Engineering and Computer Science addresses this challenge by locating fraudulent activity in the “big sea” of Medicare big data. Because identifying fraud is the first step in stopping it, this new technique could conserve substantial resources for the Medicare system.
For the study, the researchers systematically tested two large, unbalanced Medicare data sets, Part B and Part D. Part B includes coverage of Medicare medical services such as doctor visits, outpatient care, and other medical services not covered by hospitalization. Part D, on the other hand, refers to Medicare’s prescription drug benefit and covers drug costs. These data sets were tagged with the List of Excluded Persons and Entities (LEIE). The LEIE is provided by the US Office of Inspector General.
The researchers delved into the influence of random subsampling (RUS), a simple but powerful data sampling technique, and their new ensemble supervised feature selection technique. RUS works by randomly removing samples from the majority class until a specific balance between the minority and majority classes is achieved.
The experimental design investigated several scenarios, from using each technique in isolation to combining them. After the analyzes of the individual scenarios, the researchers re-selected the techniques that gave the best results and performed an analysis of the results across all scenarios.
The study results, published in the Journal of Big Data, demonstrate that intelligent data reduction techniques improve the classification of Medicare big data with high imbalance. The synergistic application of both techniques (RUS and supervised feature selection) outperformed models using all available features and data. The findings showed that the combination of using the feature selection technique followed by RUS or using RUS followed by the feature selection technique obtained the best performance.
Consequently, in the classification of any data set, the researchers discovered that a technique with the largest amount of data reduction also produces the best performance, which is the technique of performing feature selection and then applying RUS. Reducing the number of features leads to more explainable models and performance is significantly better than using all features.
“The performance of a classifier or algorithm can be influenced by multiple effects,” said Taghi Khoshgoftaar, Ph.D., lead author and Motorola Professor, FAU Department of Electrical and Computer Engineering. “Two factors that can make data more difficult to classify are dimensionality and class imbalance. Class imbalance in labeled data occurs when the vast majority of instances in the dataset have a particular label. This imbalance presents obstacles as it is possible for a classifier optimized for a metric such as accuracy to mislabel fraudulent activities as non-fraudulent in order to increase overall scores for the metric.
For feature selection, the researchers incorporated a supervised feature selection method based on feature ranking lists. Subsequently, by implementing an innovative approach, these lists were combined to obtain a conclusive feature ranking. To provide a benchmark, models were also built using all features of the datasets. In deriving this consolidated ranking, features were selected based on their position on the list.
“Our systematic approach provided greater understanding regarding the interplay between feature selection and model robustness in the context of multiple learning algorithms,” said John T. Hancock, first author and Ph.D. student in the Department of Electrical and Computer Engineering at FAU. “It’s easier to reason about how a model performs classifications when it’s built with fewer features.”
For both the Medicare Part B and Part D datasets, the researchers conducted experiments in five scenarios that exhausted the possible ways to use, or omit, the RUS data reduction and selection techniques. functions For both data sets, the researchers found that data reduction techniques also improve classification results.
“Given the enormous financial implications of Medicare fraud, the results of this important study not only provide computational advantages, but also significantly improve the effectiveness of fraud detection systems,” said Stella Batalama, Ph.D., dean of the Faculty of Engineering and Computer Science at the FAU. . “These methods, if properly applied to detect and stop Medicare insurance fraud, could substantially raise the standard of health care by reducing fraud-related costs.”
Co-authors of the study are Huanjing Wang, Ph.D., professor of computer science at Western Kentucky University; and Qianxin Liang, Ph.D. student in the Department of Electrical and Computer Engineering at FAU.
About the FAU Faculty of Engineering and Computer Science:
FAU’s Faculty of Engineering and Computer Science is internationally recognized for cutting-edge research and education in the areas of computer science and artificial intelligence (AI), computer engineering, electrical engineering, biomedical engineering, civil engineering , environmental and geomatics, mechanical engineering, and ocean engineering. Research conducted by faculty and their teams exposes students to technological innovations that drive the current state of the art in the disciplines. The university’s research efforts are supported by the National Science Foundation (NSF), the National Institutes of Health (NIH), the Department of Defense (DOD), the Department of Transportation (DOT), the Department of Education (DOEd), the State of Florida, and industry. FAU’s Faculty of Engineering and Computer Science offers degrees with a modern twist that have specializations in national priority areas such as AI, cybersecurity, the Internet of Things, transportation and supply chain management, and data science. New degree programs include Master of Science in AI (first in Florida), Master of Science and Bachelor of Science in Data Science and Analytics, and the new Master of Science and Ph.D. in computing for working professionals. For more information about the College, visit eng.fau.edu.
About Florida Atlantic University:
Florida Atlantic University, established in 1961, officially opened its doors in 1964 as Florida’s fifth public university. Today, the University serves more than 30,000 undergraduate and graduate students on six campuses located along the southeast coast of Florida. In recent years, the University has doubled its research expenditure and outperformed its peers in student achievement rates. Through the coexistence of access and excellence, FAU embodies an innovative model where traditional achievement gaps disappear. FAU is designated as a Hispanic-serving institution, ranked as one of the best public universities by US News & World Report, and a research-intensive institution by the Carnegie Foundation for the Advancement of Teaching. For more information, visit www.fau.edu.
Comments are closed.